The Ultimate Guide to Gemma Models
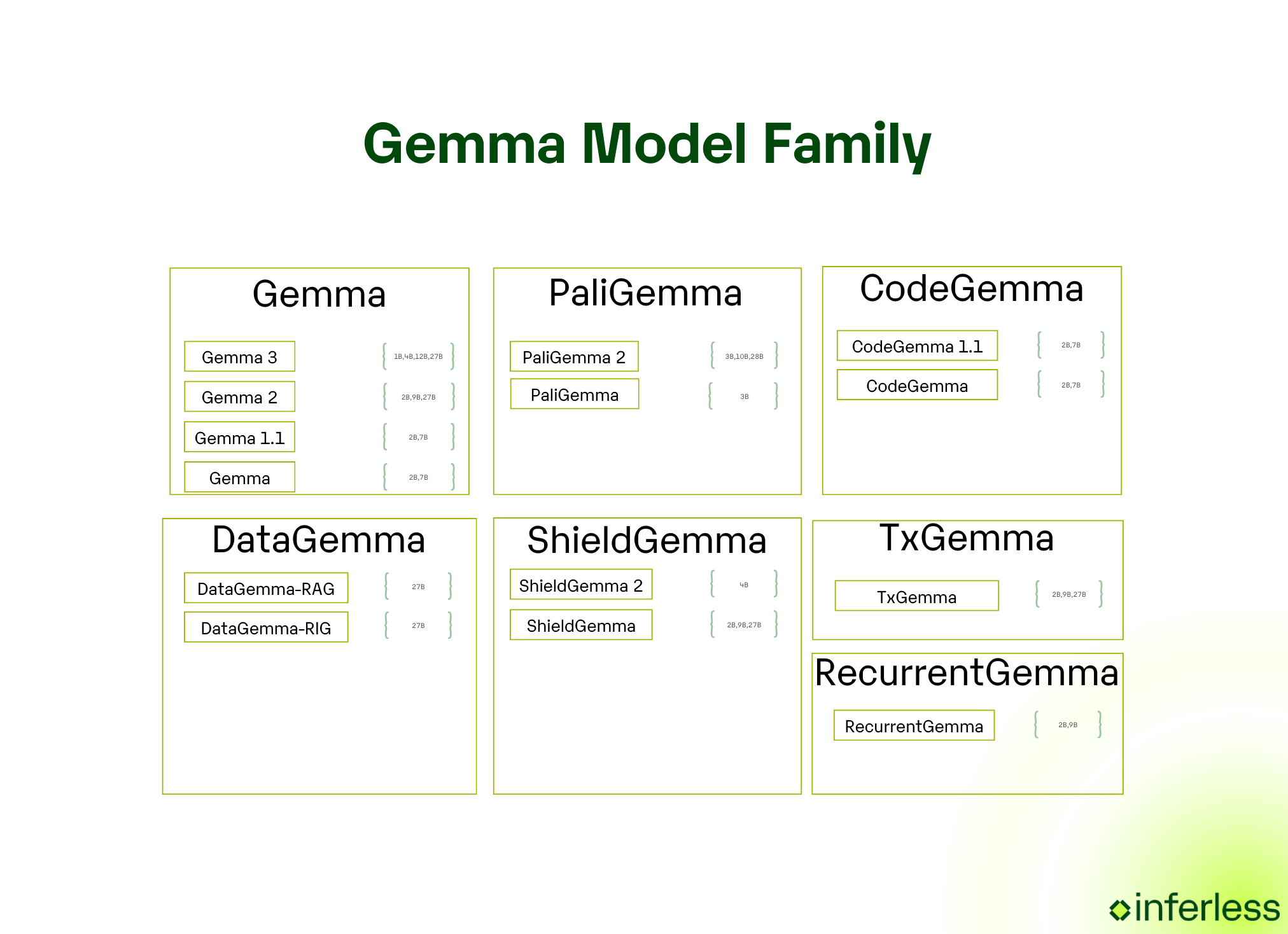
Introduction
Gemma is Google’s open family of lightweight, state‑of‑the‑art AI models, created by DeepMind and other Google teams. Built on the same research that powers the proprietary Gemini, Gemma delivers enterprise‑grade capabilities in an open format, letting anyone fine‑tune and deploy advanced models locally or in the cloud.
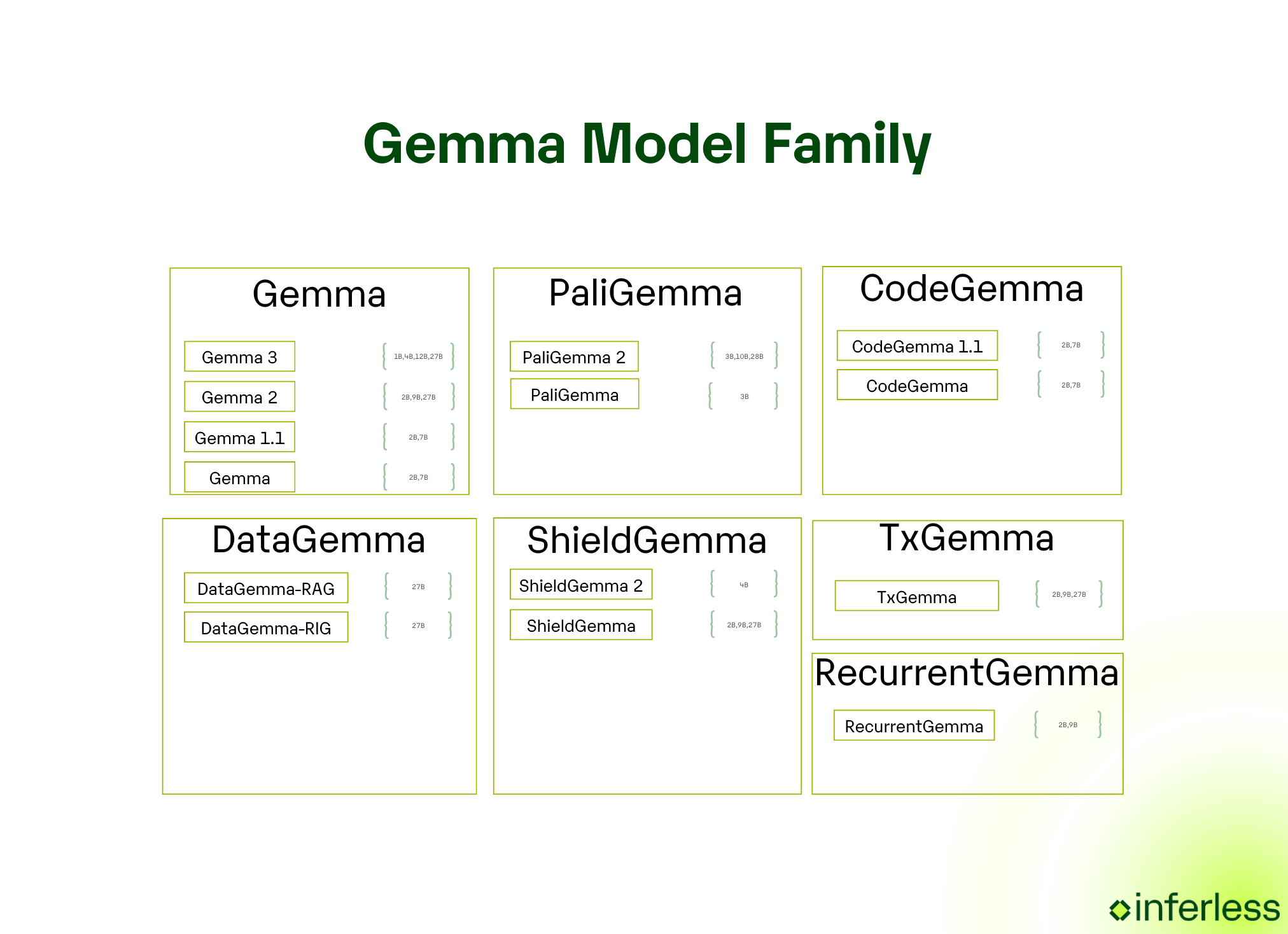
Gemma 1: The Foundation
The initial release of the Gemma family in February 2024 introduced two core models: Gemma 2B and Gemma 7B. These numbers refer to the parameter count of each model, with the 2B version containing approximately 2 billion parameters and the 7B version containing roughly 7 billion parameters. Both models were released with pre-trained and instruction-tuned variants, providing flexibility for different use cases. The pre-trained versions offered a foundation for researchers to customize through further training, while the instruction-tuned variants provided ready-to-use capabilities for direct implementation in applications.
From an architectural perspective, both Gemma 1 models utilized a dense decoder architecture with Rotary Positional Embeddings (RoPE) and a head dimension of 256. However, they differed in their attention mechanisms, with Gemma 7B leveraging a multihead attention mechanism while Gemma 2B utilized multi-query attention. This distinction was significant because the multi-query attention approach in the smaller model helped reduce memory bandwidth requirements during inference, making Gemma 2B particularly advantageous for on-device scenarios where memory bandwidth is often limited.
Gemma 2: Performance Breakthrough
Gemma 2 2B emerged as a standout model that managed to outperform significantly larger counterparts. In the LMSYS Chatbot Arena, the 2B model posts an Elo score of 1126 comfortably ahead of far larger competitors such as Mixtral‑8×7B Instruct v0.1 (1114) and GPT‑3.5‑Turbo 0314 (1106). Even models an order of magnitude larger, like Llama 2 70B Chat (1093) and the earlier Gemma 1.1 7B It (1084), trail behind.
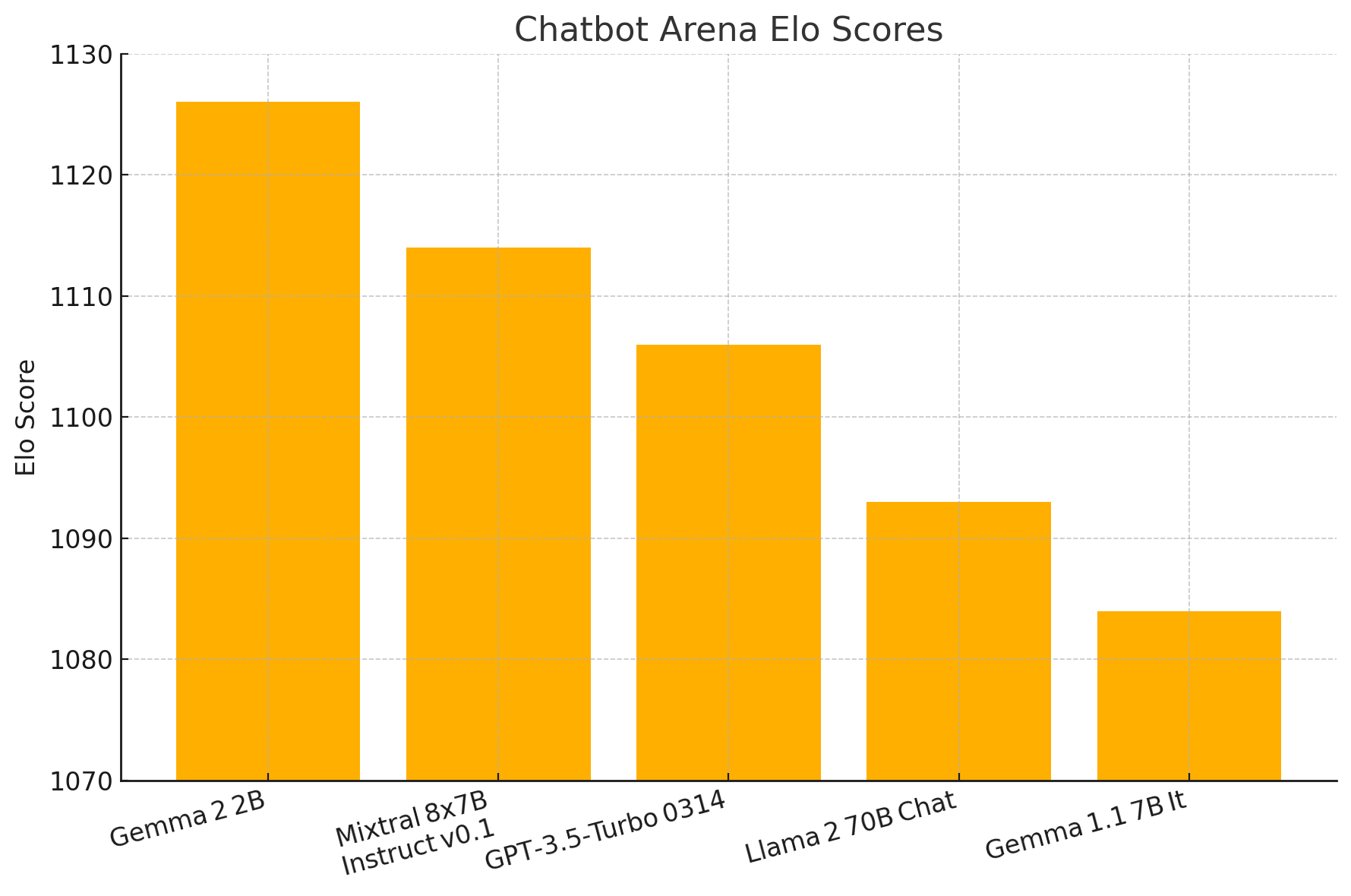
The success of Gemma 2 highlighted an important inflection point in AI model development, the recognition that parameter count alone is not the definitive factor in model performance. The improvements in Gemma 2 laid important groundwork for the more dramatic expansions that would come with Gemma 3, particularly in establishing that Google could create highly efficient models that performed above their weight class.
Gemma 3: Multimodal Revolution
Gemma 3, released in March 2025, shifts Google’s open‑model line from text‑only to truly multimodal, natively understanding images (and even short video in some variants) while expanding its context window and language coverage,, as highlighted in an in-depth analysis of multimodal models.
Gemma 3 can ingest text with images out‑of‑the‑box, with a shared vision encoder. This lets developers build apps that reason over screenshots, product photos, diagrams, or mixed media prompts capabilities absent in earlier Gemma generations, showcasing the potential of Multimodal AI within Google Cloud.
The new tokenizer and training pipeline boost coverage from mostly English to 140 + languages, support a wide variety of scripts and linguistic structures, from European languages like English and French to languages with different scripts such as Hindi and Arabic.
Another improvement in Gemma 3 is its context window size. While Gemma 1 models had a context length of 8,192 tokens, Gemma 3 extends this capability up to an impressive 128,000 tokens for most of its variants.
Gemma 3 introduced a more diverse model lineup, offering four distinct sizes to address different use cases and resource constraints:
- Gemma 3 1B: A lightweight English-only model with a 32K token context window, designed for ultra-efficient deployments.
- Gemma 3 4B: A multilingual model supporting 140+ languages with a massive 128K token context window.
- Gemma 3 12B: An enhanced multilingual model with the same, supporting 140+ languages and 128K context window but greater reasoning capabilities.
- Gemma 3 27B: The flagship model of the family, offering the most advanced reasoning and generation capabilities while maintaining the 128K context window and multilingual support.
Specialized Variants
- CodeGemma: Code‑centric Gemma (7 B pretrained, 7 B instruction‑tuned, 2 B lightweight). Trained on 500 B - 1 T code/math tokens for fast completion, generation and code‑chat. Ships with an instruction‑following version that behaves like a coding copilot out‑of‑the‑box.
- PaliGemma: 3 B vision‑language model (SigLIP encoder + Gemma decoder). Accepts images + text, supports 100 + languages, and scores strongly on captioning, VQA, detection and short‑video tasks, great for multimodal prototypes.
- RecurrentGemma: Fixed‑state, low‑memory Gemma that keeps RAM constant as sequences grow; yields higher tokens‑per‑second than standard Transformers, so you can run very long‑context experiments on a single GPU or even a CPU.
- DataGemma: Gemma tied to Google Data Commons. Uses RIG/RAG to fetch live statistics before and during generation, grounding answers in 240 B trusted data points, ideal for dashboards, policy briefs and anything numeric.
- TxGemma: Drug‑discovery suite (2 B/9 B/27 B “predict” models). Trained on 7 M therapeutic examples; SOTA on 64/66 property‑prediction tasks and competitive with single‑task specialists, potentially shaving months off early‑stage R&D.
- ShieldGemma: Safety guardrails (2 B/9 B/27 B). Moderates sexually explicit, dangerous, hateful and harassing content in both user prompts and model outputs, and is released with permissive open weights for easy integration into any Gemma‑based stack.
Core Architecture
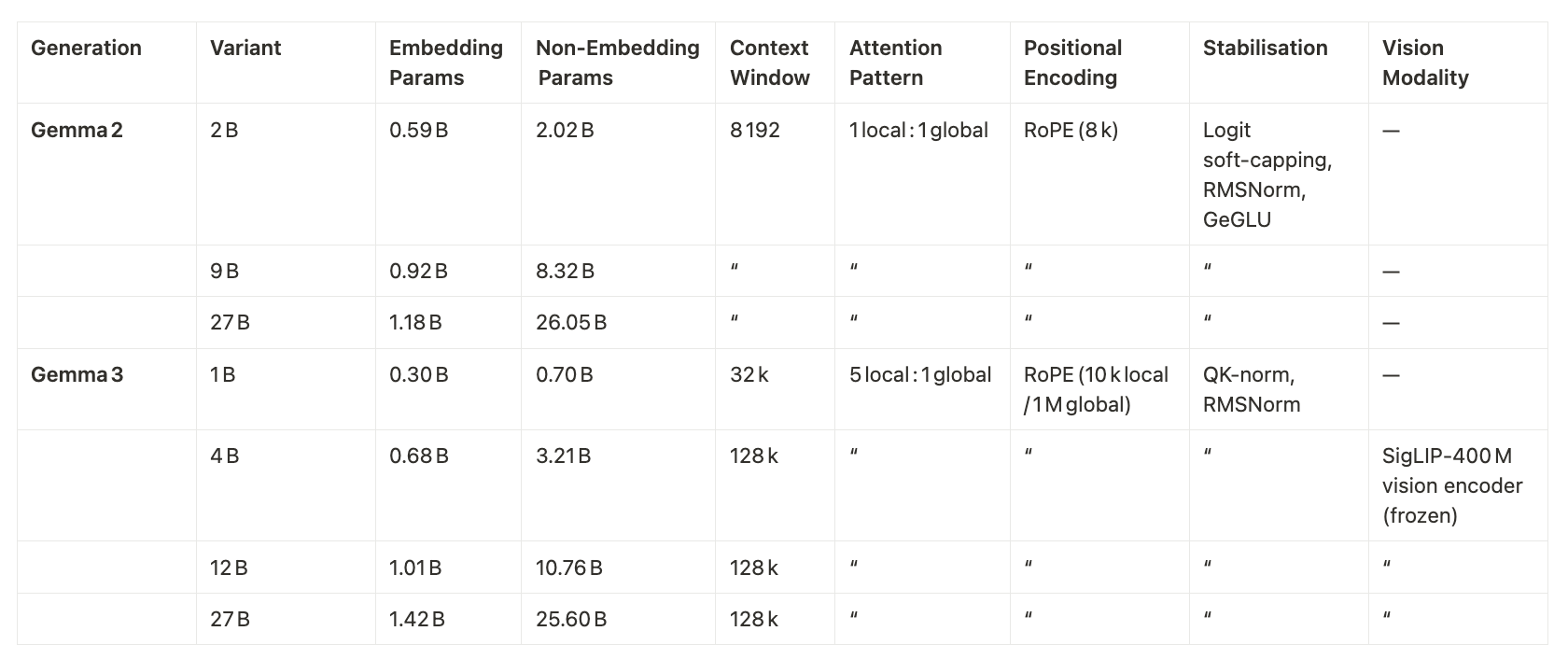
Gemma’s design stays true to the “decoder‑only Transformer” recipe, but each generation tweaks it for longer context and lower cost.
Gemma 2:
The model can read up to 8 000 tokens at once. Inside every pair of layers, one layer looks only at a sliding 4 000‑token window (cheap) while the next can see the full 8 000‑token span (expensive but rare). Positions are handled by rotary embeddings; activations are kept stable with RMSNorm, a GeGLU feed‑forward block, and a tanh “soft‑cap” that clips extreme logit values. Grouped‑Query Attention (two query groups) further speeds inference.
Gemma 3:
It keeps the same backbone but makes three clear upgrades:
- Much longer context. Most sizes now handle 128k tokens (32 k for the 1 B model) by raising the rotary frequency for global layers and interpolating positions.
- Cleaner normalisation. The old logit clip is replaced with QK‑norm, a lighter‑weight way to stop values from blowing up.
- Smarter attention schedule. Five cheap local layers are followed by one global layer, giving strong local reasoning without losing document‑level awareness.
Gemma 3 also becomes multimodal. A frozen 400 M‑parameter SigLIP vision encoder turns 896 × 896 images into embeddings; when pictures are oddly shaped, a “Pan & Scan” step tiles and resizes them so nothing important is lost. Those image tokens are simply fed into the same language stack.
Gemma Training
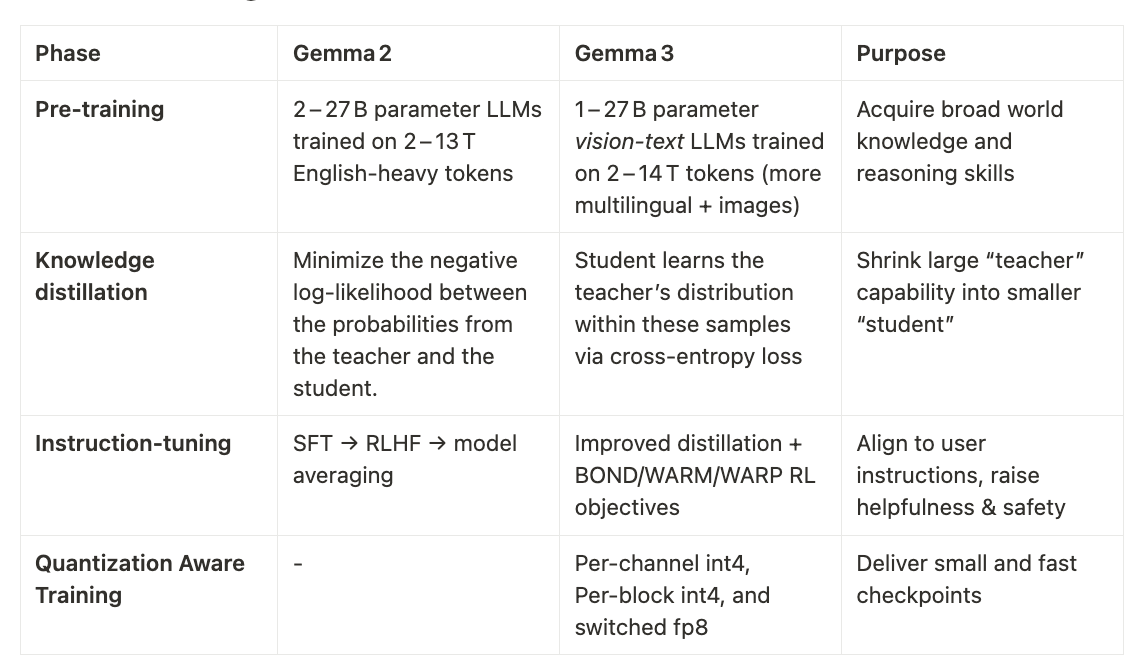
Gemma models are first trained on trillions of tokens drawn from web pages, code bases, scientific articles and, for Gemma 3, large‑scale image–text pairs plus an expanded multilingual corpus, which provides a foundational understanding of multimodality. Text is processed with a SentencePiece tokenizer (256 k vocabulary in Gemma 2, 262 k in Gemma 3) that preserves white‑space, splits digits and operates at the byte level; every sample then passes a multi‑stage filter that strips personal data, unsafe content, evaluation leaks and low‑quality passages, the latter re‑weighted in Gemma 3 to favour higher‑grade sources.
Pre‑training itself is formulated as knowledge distillation: each student network minimises the cross‑entropy between its predicted token distribution and that of a much larger teacher. In Gemma 3 the cost is reduced by sampling 256 logits per position and renormalising the teacher distribution over that subset.
After convergence the raw checkpoints are converted into instruction‑tuned variants. The pipeline begins with supervised fine‑tuning on synthetic and human prompt‑response pairs whose answers are mostly generated by the teacher; it continues with reinforcement learning from human feedback, where a reward model an order of magnitude larger than the policy steers optimisation toward helpfulness, factuality and safety.
Finally, each checkpoint is re‑released in several quantisation formats.
Evaluation and Performance
Instruct Performance:
Across the standard instruction tests, Gemma 3‑it (27 B) delivers a balanced result. Its general‑knowledge scores on GPQA Main/Diamond (36.8 / 42.4) and MMLU (76.9) sit just behind similarly sized models but it matches them on coding (HumanEval 87.8) and clearly leads the mathematical reasoning, posting an exceptional 89 % on the MATH benchmark which is almost twenty points higher than the next best model.
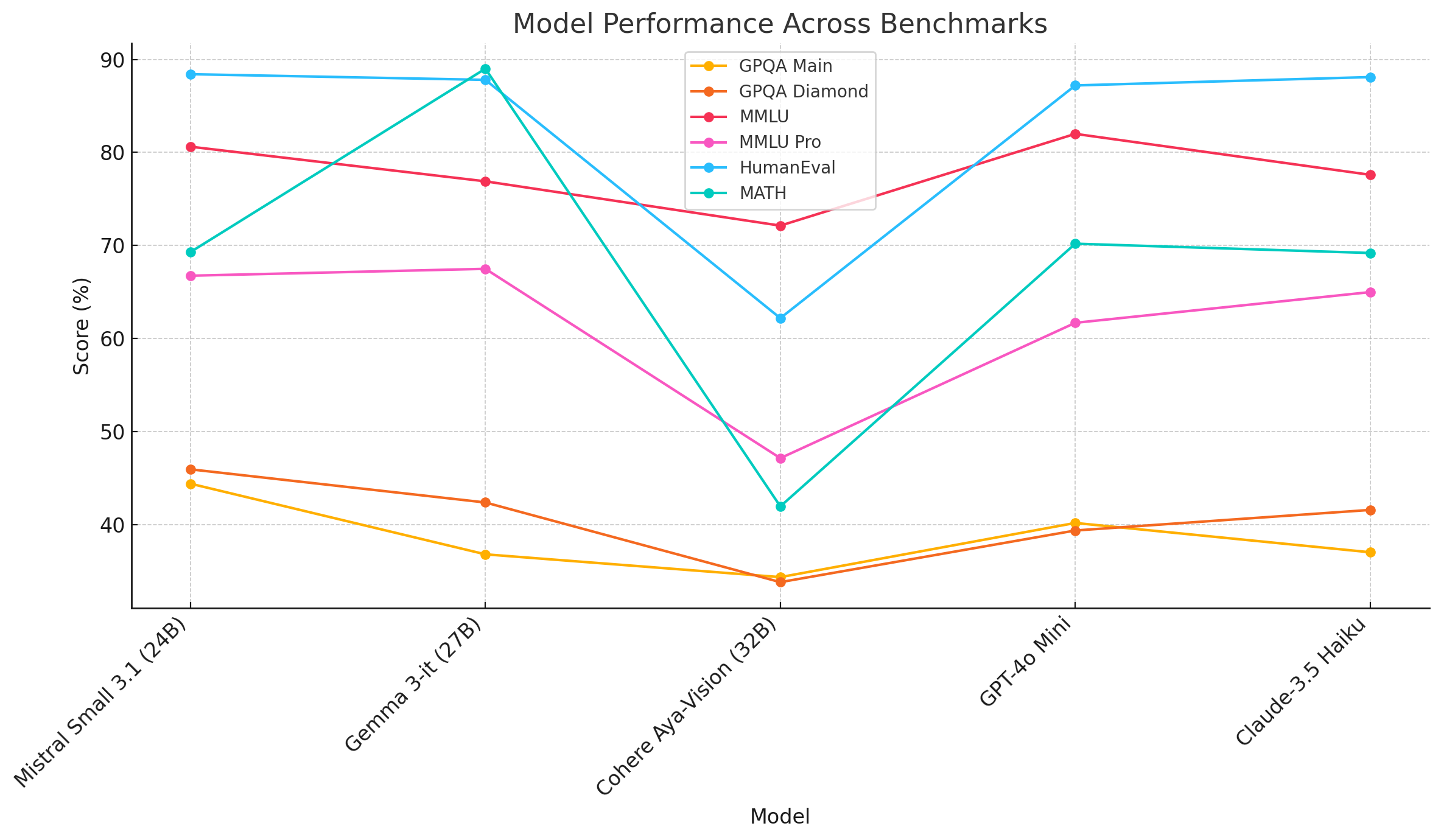
Multimodal Benchmark
On vision, Gemma 3 remains competitive as it just less then a point behind Mistral on MMMU‑Pro, stays within a point on MathVista, and actually tops on the MMMU (64.9).
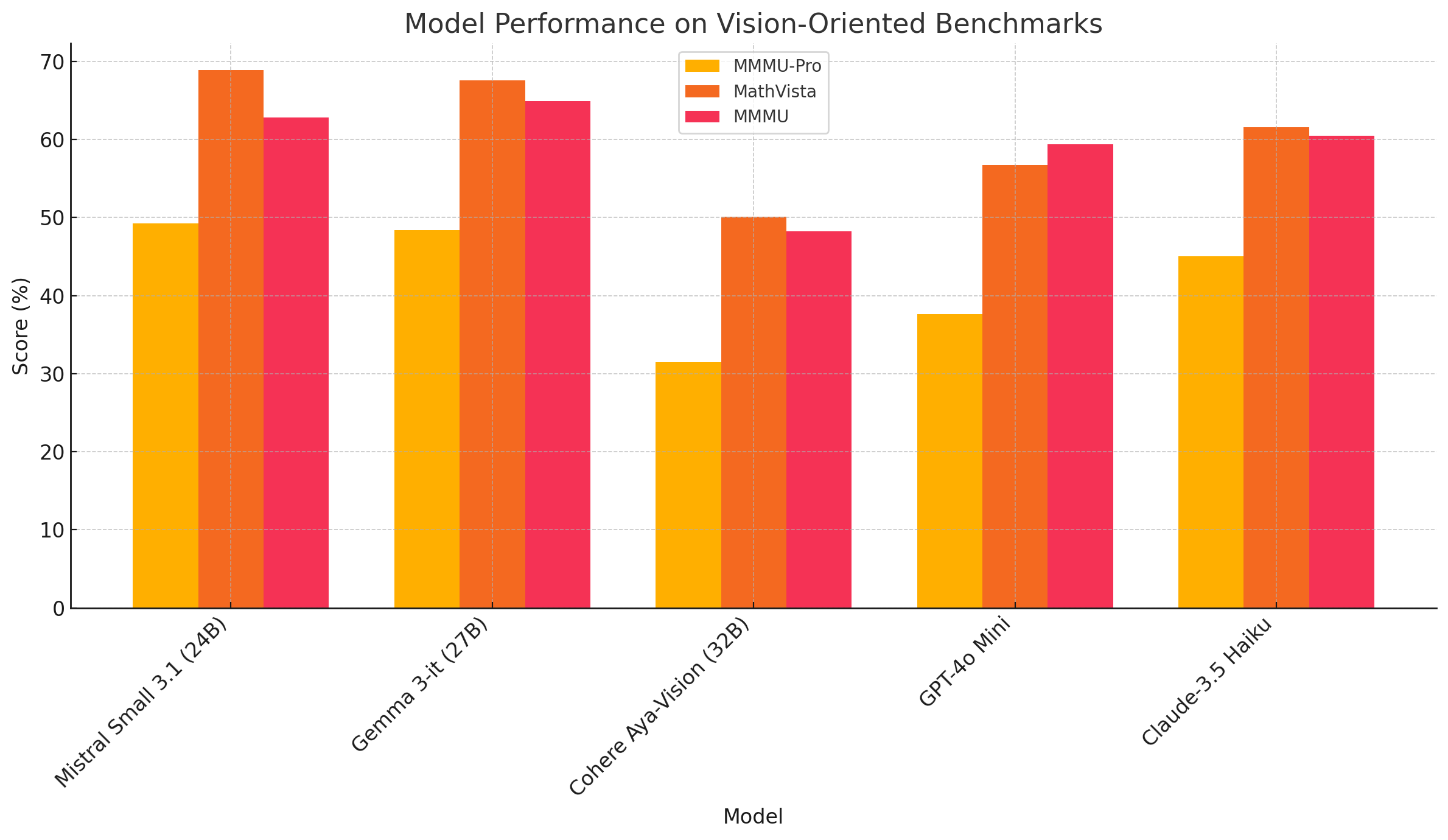
Multilingual Support
Gemma 3 has been trained on a pretraining dataset that now includes twice the multilingual data compared to previous iterations. By carefully revisiting the data mixture and integrating both monolingual and parallel data, this model achieves robust coverage of over 140 languages. This strategic data enhancement enables the model to capture the nuances of both high-resource and low-resource languages, ensuring more reliable and inclusive performance worldwide.
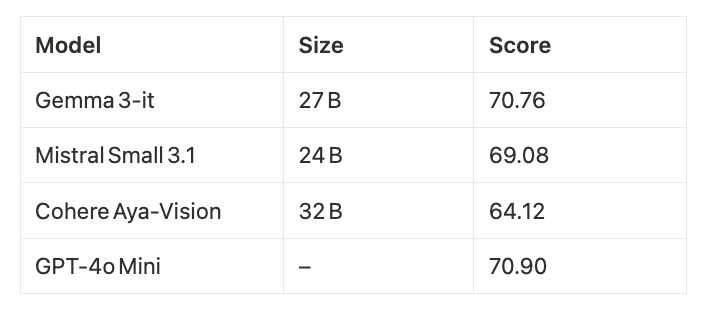
The tokenizer has been refined to better encode languages with complex scripts such as Chinese, Japanese, and Korean, even if it marginally increases token counts for English and code. The result is a balanced token representation that significantly improves multilingual comprehension and generation.
The result is a model that matches GPT‑4o Mini and surpass other models on Middle‑Eastern benchmark while maintaining top‑tier scores elsewhere.
Gemma Model Use Cases
Gemma make it easy to slot the model into everyday products. The examples below show how developers are already using Gemma into production workflows.
1. Conversational Agents & Customer Support
Lightweight instruction‑tuned checkpoints (e.g., gemma-3-1b-it or gemma-2-2b-it) power chatbots that run in a browser tab or directly on edge GPUs for serverless GPU inference. They handle FAQ lookup, order status questions, or escalation routing with latency low enough for live chat.
A Google Cloud notebook shows how Gemma streams support tickets, classifies sentiment, and drafts immediate replies in near‐real‐time a template for contact‐center automation.
2. Knowledge Retrieval & Question Answering
With context windows up to 128K tokens in Gemma 3, you can stuff entire policy manuals or research papers into the prompt and ask natural‑language questions, eliminating database schema work.
Developers have paired Gemma with in‑browser vector stores to build on‑device RAG assistants that never leave the user’s machine, ideal for corporate compliance or offline fieldwork.
3. Code Generation & Developer Productivity
For developers, tools like Continue (the open‑source autopilot for VS Code and JetBrains) can be paired up with Gemma 3 through Ollama so you get inline completions and refactors without sending code to the cloud.
4. Browser Extensions:
Lightweight extensions bring Gemma right into the browser. The open‑os LLM Browser Extension is “compatible with any Ollama so users can open a sidebar chat or hover‑translate text using your local Gemma model.
Gemma: Community and Ecosystem
Since its launch in February 2024, Google’s open‑source Gemma model family has attracted a fast‑growing network of developers, researchers, and companies.
The ecosystem revolves around the Gemmaverse, a vibrant community space showcases community-created model variants and implementation case studies, serving as both inspiration and practical resource for newcomers and experienced developers alike.
The Gemma community actively engages across several platforms, with particularly notable activity in dedicated Discord channels. Google Developer's #gemma Discord channel has become a hub where developers can chat in real-time, exchange ideas, troubleshoot implementations.
Beyond Discord, the Kaggle platform hosts a significant Gemma community presence, offering interactive notebooks, model cards, and community discussions, complementing valuable resources for AI/ML applications.
For hands‑on guidance, Google maintains the repo on GitHub, a concise set of notebooks and code snippets that walk users through training, fine‑tuning, and deployment with cost-effective options available. Together, these resources keep the Gemma ecosystem moving quickly from concept to production.
Step-by-Step Guide for Inference of Gemma-3 Models
This guide provides a comprehensive approach to deploying and running inference with Google's Gemma-3 models using the vLLM framework.
Prerequisites
- Python Environment: Ensure you have Python installed (preferably Python 3.8 or later)
- Install Required Packages: Install the required libraries using pip:
pip install vllm==0.8.4 transformers==4.51.3
- Hugging Face Account: You'll need to accept the Gemma-3 license on Hugging Face to access the models
Step 1: Initialize the Tokenizer and Model
Begin by importing necessary libraries and initializing the tokenizer and model.
from vllm import LLM
from vllm.sampling_params import SamplingParams
from transformers import AutoTokenizer
import os
os.environ["HF_TOKEN"]="<YOUR_HUGGING_FACE_ACCESS_TOKEN>"
# Define the Model name (choose one based on your needs)
model_id = "google/gemma-3-4b-it"
# Initialize the tokenizer
tokenizer = AutoTokenizer.from_pretrained(model_id)
# Load the model
llm = LLM(model=model_id)
# Set up sampling parameters for generation
sampling_params = SamplingParams(
temperature=0.7,
top_p=0.9,
repetition_penalty=1.1,
max_tokens=512,
top_k=40
)
Step 2: Prepare Your Prompt
Define the prompt for generating responses from the model.
# Prepare your prompt
prompt = "Explain the concept of transfer learning in AI."
# Gemma-3 supports chat format
messages = [
{"role": "user", "content": prompt}
]
Step 3: Generate Responses
Use the LLM
instance to generate responses based on your prepared messages.
# Apply the chat template to format the messages correctly for Gemma-3
input_text = tokenizer.apply_chat_template(messages, tokenize=False)
# Generate response
response = llm.generate(input_text, sampling_params=sampling_params)
# Extract and print the generated text
generated_output = response[0].outputs[0].text
print(generated_output)
Step 4: Using Quantized Models for Efficiency
If you're working with limited GPU resources, you can use quantized versions of Gemma-3 models.
# For GPTQ quantized models
llm = LLM(model="ISTA-DASLab/gemma-3-27b-it-GPTQ-4b-128g")
Now you can just follow the same process to use the Quantized model.
Conclusion
Google's Gemma family represents a remarkable evolution in open-source AI models, transforming from a text-only foundation to a comprehensive multimodal system in just over a year. What began as an efficient alternative to proprietary models has rapidly matured into a versatile AI ecosystem that challenges much larger systems while maintaining accessibility.
Perhaps most impressive is how Gemma has expanded beyond a single model from CodeGemma for developers to TxGemma for pharmaceutical research.
AI continues to evolve, Gemma's open approach stands as a counterbalance to closed, proprietary systems, as discussed in the Inferless blog on model inference. As the family continues to grow and mature, it seems poised to play an increasingly central role in bringing advanced AI capabilities to more users worldwide, reflecting the trends discussed in a comprehensive overview of multimodal AI.
Resources:
[1] https://ai.google.dev/gemma/docs/codegemma/model_card
[2] https://ai.google.dev/gemma/docs/paligemma/model-card
[3] https://adasci.org/a-hands-on-guide-to-recurrentgemma-with-huggingface/
[4] https://www.maginative.com/article/google-introduces-datagemma-to-improve-ai-accuracy-with-trusted-data-sources/
[5] https://developers.googleblog.com/en/introducing-txgemma-open-models-improving-therapeutics-development/
[6] https://build.nvidia.com/google/shieldgemma-9b/modelcard
[7] https://callchimp.ai/blogs/understanding-google-gemma
[8] https://www.linkedin.com/pulse/building-sota-ai-gemma-3-extended-context-support-seamless-anand-bmw1c
[9] https://developers.googleblog.com/en/gemma-family-expands-with-models-tailored-for-developers-and-researchers/
[10] https://developers.googleblog.com/en/gemma-explained-paligemma-architecture/
[11] https://www.searchenginejournal.com/recurrentgemma/514392/
[12] https://ai.google.dev/gemma/docs/datagemma
[13] https://www.infoq.com/news/2025/03/txgemma-google-deepmind/
[14] https://ollama.com/library/shieldgemma
[15] https://sushant-kumar.com/blog/gemma
[16] https://www.reddit.com/r/SillyTavernAI/comments/1i794qk/kv_cache_size_of_each_model_per_token/
[17] https://ai.google.dev/gemma/docs/codegemma
[18] https://ai.google.dev/gemma/docs/paligemma
[19] https://ai.google.dev/gemma/docs/recurrentgemma
[20] https://huggingface.co/google/datagemma-rag-27b-it
[21] https://www.datanami.com/this-just-in/google-announces-gemma-a-new-family-of-open-ai-models-inspired-by-gemini/
[22] https://cloud.google.com/blog/products/ai-machine-learning/performance-deepdive-of-gemma-on-google-cloud
[23] https://meetcody.ai/blog/gemma-2-2b-architecture-innovations-and-applications/
[24] https://dev.to/sathish/gemma-3-deepminds-leap-in-multimodal-ai-jgb
[25] https://dev.to/_37bbf0c253c0b3edec531e/what-is-gemma-3-1fg
[26] https://dev.to/best_codes/gemma-3-googles-open-multimodal-model-with-long-context-vision-49c3
[27] https://siliconangle.com/2024/02/21/google-releases-gemma-family-open-source-ai-models-inspired-gemini/
[28] http://arxiv.org/pdf/2406.11409.pdf
[29] https://www.marktechpost.com/2024/06/27/google-releases-gemma-2-series-models-advanced-llm-models-in-9b-and-27b-sizes-trained-on-13t-tokens/
[30] https://developers.googleblog.com/en/introducing-gemma3/
[31] https://www.youtube.com/watch?v=ziPBCrNStdk
[32] https://www.youtube.com/watch?v=Be4yYekHFRs
[33] https://www.geeky-gadgets.com/google-gemma-3/
[34] https://blog.google/technology/developers/gemma-3/
[35] https://blog.gopenai.com/gemma-3-googles-revolutionary-multimodal-ai-model-reshaping-the-future-of-artificial-intelligence-d60bc22b05d8?gi=efe459410036
[36] https://ai.google.dev/gemma/docs/model_card
[37] https://blog.paperspace.com/gemma/
[38] https://ollama.com/blog/gemma2
[39] https://www.bizzbuzz.news/technology/ai/google-unveils-gemma-3-ai-models-with-multimodal-capabilities-1355482
[40] https://blog.google/technology/developers/google-gemma-2/
[41] https://www.promptingguide.ai/models/gemma
[42] https://developers.googleblog.com/en/gemma-explained-new-in-gemma-2/
[43] https://blog.roboflow.com/gemma-3/
[44] https://blog.google/technology/developers/gemma-open-models/
[45] https://arxiv.org/html/2406.11409v1
[46] https://www.neowin.net/news/googles-gemma-2-delivers-powerhouse-performance-at-a-fraction-of-the-cost/
[47] https://www.geeky-gadgets.com/google-gemma-3-multimodal-ai-model/
[48] https://currentaffairs.adda247.com/google-unveils-gemma-3-the-next-evolution-in-lightweight-ai-models/
[49] https://www.storagereview.com/news/google-gemma-3-and-amd-instella-advancing-multimodal-and-enterprise-ai
[50] https://www.classcentral.com/course/youtube-gemma-3-435930
[51] https://www.techtarget.com/searchenterpriseai/definition/Gemma
[52] https://www.linkedin.com/posts/merve-noyan-28b1a113a_gemma-3-can-understand-videos-and-its-more-activity-7305589304490139649-KjZE
[53] https://ai.google.dev/gemma